Founder of Constellation Research & Author, Ray Wang, says that machine learning and AI are helping "To streamline information so that we can get the right information at the right time in the right mode, ... when you need it." And Information Week just declared, "AI, Machine Learning Rising In The Enterprise".
We have also got our machine learning hands dirty with team Clear Metal who will soon unveil how they are using predictive data science to solve the most complex operations challenges in container shipping. Stay tuned to learn more about how Big Data Meets Big Metal with the new Clear Metal predictive logistics platform.
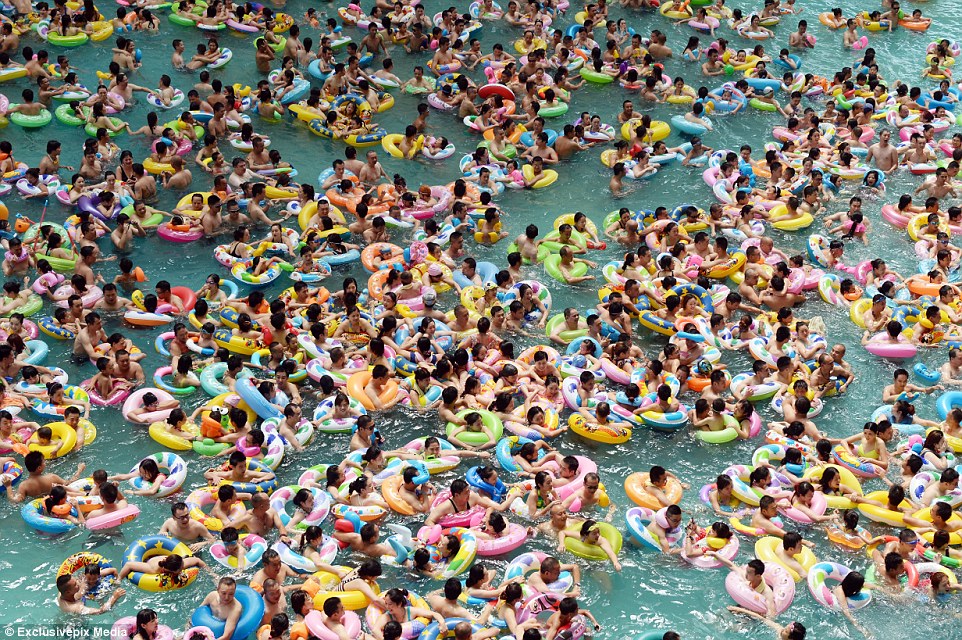
Often when a new technology ascends, it holds the promise of creating a big, new growth market with massive category potential. Once this fire gets stoked it's as if someone stands up in the middle of Silicon Valley with a blow horn shouting, "Everyone into the pool!"
Startups pop up, venture capitalists crack open their coffers and large existing players re-position their products while scrambling to build or buy new technology, all in an effort to capture the promise of new category potential.
Today the most powerful transforming force in business is the category. Said another way, the category is the "pool" that entrepreneurs and venture capitalists cannon ball into! As you know, there is no such thing as a hot company in a cold space. New categories are where people look for new growth opportunities. As a result, the entire tech world is always new category hunting. We've found a link, based on data science research, between category evolution and technology company value creation.
The January-February issue of the Harvard Business Review featured a story based on our research into how technology startups create enduring value. The article highlights the discovery of an IPO Sweet Spot that identifies where the value is created for technology IPOs. Specifically, we discovered that companies that go public between 6.5 and 10.5 years old create the vast majority of post-IPO value. You can get the full report here: Play Bigger IPO Sweet Spot Report.
We also know that new categories go through three main stages: define, develop and dominate. The research of Paul Geroski, author of The Evolution of New Markets, charts this path. In a category's earliest stage, the number of companies in the space explodes. This is the phase when the new category is first designed and a gaggle of entrants are scrambling to leverage new technology to solve new or existing problems in innovative ways.
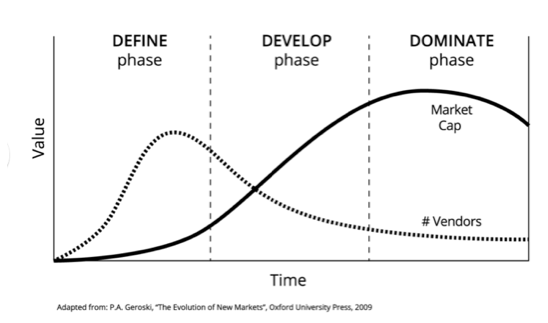
In the middle phase, the number of companies consolidate as a category king emerges and competitors disappear while the category king sucks up 76% of all the economics. In the last phase, the number of companies trickle away as the king dominates and reigns over the market.
When you connect the dots between the evolution of new categories and the IPO sweet spot, it seems to show that the best time for a company trying to design and dominate a new space to go public and the moment of category explosion are the same - in the develop stage. And in the post-Internet era, that's consistently been about six to ten years after the first companies are founded in the category.
So what does all of this mean to the frothing machine learning space? If you assume that the average machine learning startup is plus or minus one to two years old, then we will see machine learning companies going public in about five to seven years in an effort to create enduring value.
Now all of this prognostication is predicated on the huge assumption that machine learning will power new market categories. If the experts are right, this new technology will opens doors to new market opportunity. We're not in the forecasting business, so we'll leave that up to the gurus. It seems that a lot of entrepreneurs, VCs and big existing tech players are placing their bets now. What we do know, based on data science, is that so far in this century the companies that create the most post IPO value do it within the 6.5 to 10.5 year sweet spot. Which tells us, in five to seven years we'll all know if the machine learning seeds being planted today will bear fruit.
IPO Sweet Spot
The response to the IPO Sweet Spot research in the Harvard Business Review drew a lot of attention and commentary. A lot of folks asked us to take new cuts at the data such as different time frames and industries. This has inspired us to keep our data team working hard and you can expect to see more from us this year.
We also have to thank our friends for tweeting and spreading the word about the IPO Sweet Spot. Specifically, tweets from Bill Gurley at Benchmark and Ann Miura-Ko at Floodgate about blew our website off its axils.
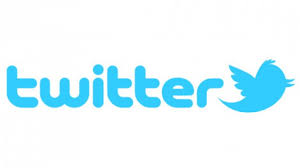
Going Long
Glen Solomon, partner at GGV Capital gave an interesting take on the IPO Sweet Spot in his recent blog.
"Founder's have a lot on their plates, but one of their most important jobs is to become a ninja category designer, making sure they've built a solution to address and win a very large market. As Peter Thiel notes in Zero to One, some successful founders start with a small market and find ways to grow it to something much larger over time. The key is the size of the ultimate market, not its initial size."
Why These Companies Were Hot In 2015
And why others were not.
On a different topic, Chris took a look back at 2015 and asked "What makes one company hot and another, well, not?" Check out Christopher's thoughts on the rise of Atlassian, the swirling sound stalking Yahoo!, and Facebook's non stop successes.